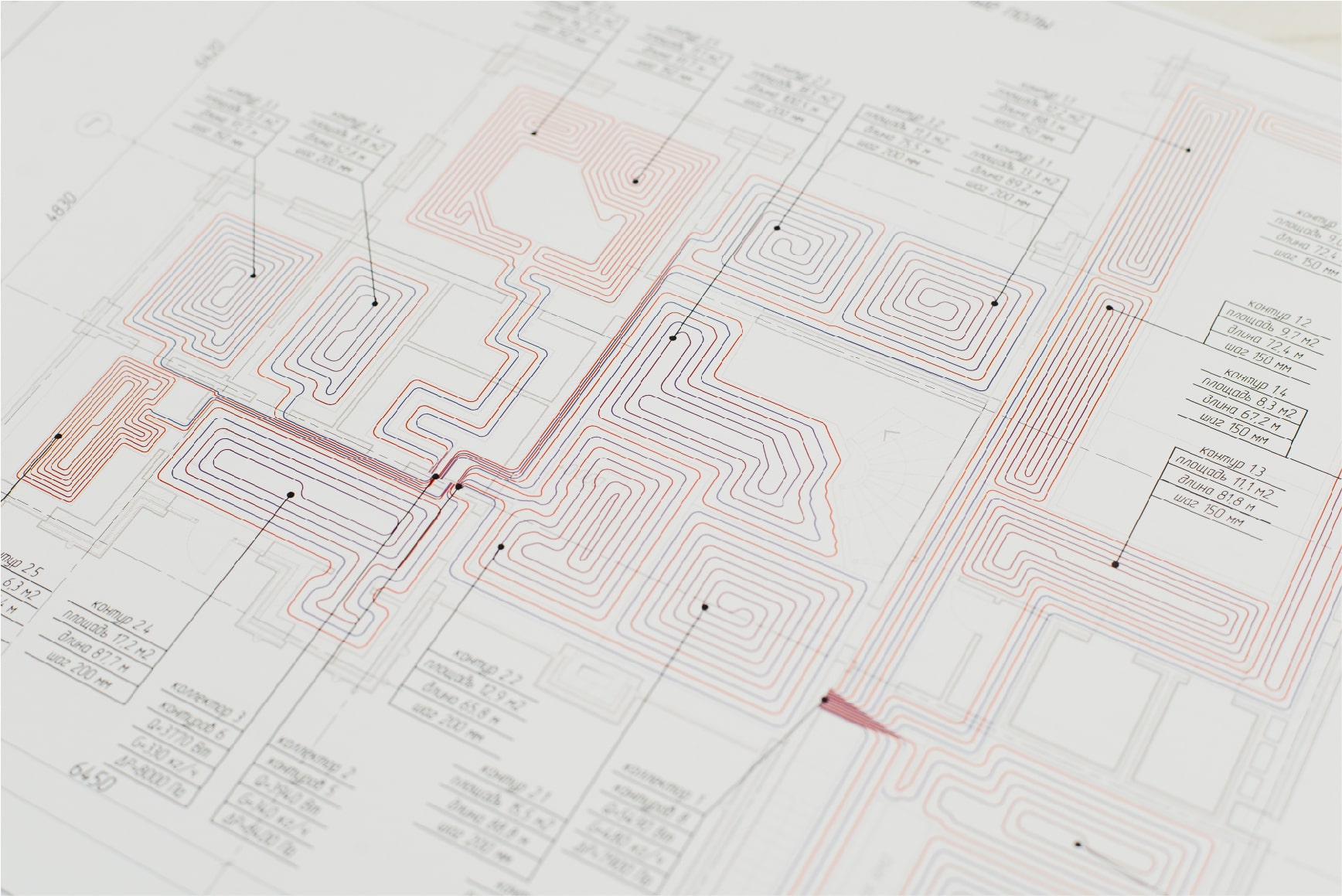
Neural networks in analytical chemistry
What this post is about
Neural networks (NNs) have become one of the most popular and relevant areas of research of machine learning (ML). A NN is a series of algorithms intended to recognize patterns within datasets. Applications range from sales forecasting, customer research, data mining to recognizing pictures and require lots of data to determine underlying relationships. Likewise, routine analyses in analytical chemistry generate considerable amounts of data in the form of spectra etc. Nonetheless, NNs are not frequently encountered in this field. This blogpost provides a brief explanation of a NN and highlights three recent successes of the application of NNs in chemistry.
What is a neural network?
The name ‘neural network’ is derived from the similarity to the brain, composed of a complex architecture of neurons. The neurons in a neural network are connected via strings called ‘edges’. In simple NNs, there is a layer of input neurons that accept data, and a layer of output neurons that contain the outcome of the process. In between the two, are one or more ‘hidden layers’, layers of neurons that connect the input and the output neurons. Each neuron in the network accepts a signal from a neighbouring neuron, processes it, and sends the signal to another neuron. The strength of the signal can be increased or decreased by the ‘weight’ of the edge or neuron, a value that can be changed between iterations to refine the outcome of the model.
The theory behind: In a nutshell
Atoms and bonds can be represented by a set of nodes and edges, respectively. MIT researchers have used this representation to train a NN in assessing the chemical reactivity of a pool of organic molecules.1 Surprisingly, their approach predicts the major product correctly in 85% of the cases, on par with expert organic chemists. In a completely different approach, scientists from Japan used a type of NN to classify the metabolic profiles of fish captured in 1H NMR spectra to predict their origin.2 Their approach led to correct classification in 97.8% of the cases, and additionally was able to identify compounds that were characteristic for fish from a specific origin. Besides 1H NMR spectra, IR spectra have also been used for the classification of species.3 A group of researchers used FT-IR hyperspectral imaging in combination with a NN to identify pathogenic bacteria. The resolution of their method was high enough to extend below the level of individual bacterial species.
No NN without coherent data
The applications mentioned above demonstrate the great promise that ML brings to the scientific community. We can imagine that within a decade, ML is a routine method to aid in the analysis of compounds and mixtures. But for that to happen, the data needs to be generated and stored in a coherent way. For smaller analytical studies, the development of large databases of spectra will tremendously aid in the implementation of ML for chemical analysis. Without a doubt, NNs will continue to become more powerful and have an immense impact on the way we process information in the future.
We can help you make the most of your data
Together with the group AI4Science at the University of Amsterdam we are always on the lookout for cases where we can perform predictive ML on your datasets. Get in touch with us to learn more on how.
(1) Coley et al., Chem. Sci. 2019,10, 370-377; (2) Date, Kikuchi, Anal. Chem. 2018, 90, 3, 1805-1810; (3) Lasch et al., Anal. Chem. 2018, 90, 15, 8896-8904
Let's
talk.
Together with you, we
make sure that we find
the answers.
Call us on +31 6 27080833
or use the form.
